Questions we’re asking of AI startups in 2025.
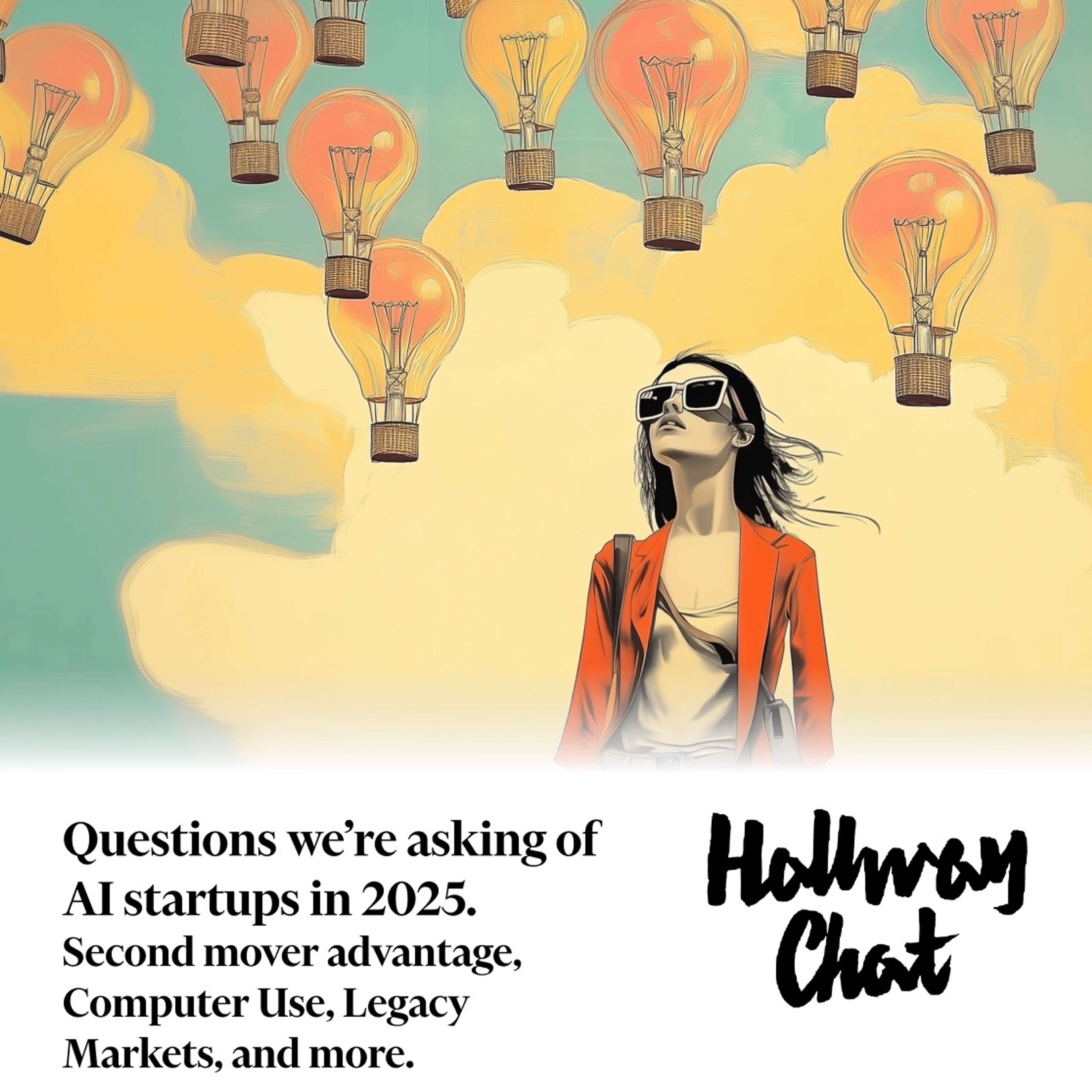
Today, Nabeel and Fraser tackle the questions they've been asking internally. What level of improvement would it take to disrupt an incumbent product? They also explore what advantages second movers have in product markets, the emerging importance of reasoning models and computer use products, and what makes certain legacy markets ripe for AI reinvention.
[00:00:00]
Fraser: We are seeing everybody do the low hanging fruit first, right? Like, all variations of deep search or deep research are the obvious first thing to be
Nabeel: doing. Once they've picked their toothpaste, like, you're not switching them off their toothpaste. But when toothpaste comes out for the first time, you go through this period where you're like, Well, what kind of toothpaste am I supposed to use?
Whatever is broken often is the first step to leading to a new solution.
Fraser: You, you think, you think if Grok is 10 percent better than ChatGPT, you thought that if it remained 10 percent better, it could win. Mm
Nabeel: hmm.
Fraser: I can't. I can't get my head around that. And I'm so baffled by it that I know that you and I must be talking about two different things.
I so strongly disagree with it that we must just be talking like we're making different assumptions about what we're talking about. Or why don't we just handle it right now? And that'll be your opening. Yeah. Well, welcome. Welcome back, everybody.[00:01:00]
Welcome to
Nabeel: hallway chat. I'm Nabeel.
Fraser: I'm Fraser.
Nabeel: That's the way to keep it loose.
Just get in the middle of it.
Fraser: You dropped that on me when I had to run and that's been bouncing around my head since you did it. I so strongly disagree with you that my only conclusion is that we're making different assumptions. And so you got to set it up and tell me your assumption here.
Nabeel: I liked your phrasing.
My assertion was that if an LLM model that we're using today, so particularly a chat based model, let's talk about ChatGPT, Grok, Claude, DeepSeek, or whatever, you know, that has some measure of traction that it's in the comparison set. Let's just say that. But what I actually said was, if the product is 10 percent better, it's going to win.
That it doesn't need to be two times better or 10 times better. Like, if it's 10 percent better, it's actually going to win over the longer arc. What's wrong with that? Doesn't the better product win, Frasier?[00:02:00]
Fraser: No, no, like, ChatGPT has 400 million weekly active users now. They have Escape Velocity, they have the brand, they have the trust, they're like a global entity. And if you're always just 10 percent better, I can't imagine there's any hope in hell that you catch up. Like, I just can't imagine, like, I can't, the, the share of users that care about a 10% Improvement in that product is got
Nabeel Hyatt: to be so small.
We might just disagree. We might actually disagree with this one.
Nabeel: So, but, but,
Nabeel Hyatt: but I think there are definitely a couple of assumptions. So like, if you were advising a founder
Nabeel: who was always a competitor to player in the space, you would advise that you could win at the model game by going after some other affordance you
Nabeel Hyatt: hit.
It checks PT where it's weak, go after some area where it's that you're 10 times better. And I have to be honest, like, obviously, most of my life when I'm talking to a founder saying the same thing, saying like, listen, you just [00:03:00] can't be a little bit better. Like that's how startups die is that they find some minor optimization arbitrage in the world and think that that's going to be enough.
And like, people don't care. And so you, you're not 10 X, but it's funny when I. You know, we're, we're generalists at Spark and we do all kinds of different investing. I find that that's a struggle to try and explain to these like seed and pre seed and angel investors who are like, what are you into now?
And like, everybody speaks in these, these stupid VC, you know, invented market maps and verticals and stuff like that, which ironically we're about to do in a minute. It's like, let's talk about these as categories when really the most interesting companies are the ones that are inventing new categories that we hadn't thought of before that those are the places that get really excited.
But one of the ways I talk to somebody at a pre seed fund, and I'm like, oh, what company should I go talk to you about? It's like, well, don't bring me the things that are like 10 percent better. Bring me, like, if it just like, you open up the product and you use it, and even if it's in its early and mildly broken form, you're just like, oh my God, like there's a real [00:04:00] like bolt of lightning.
It's 10x better at one thing, even though it's maybe worse in lots of other things. Like that's the thing to bring to me. So you're right. And there's some cognitive distance between that and me saying what I said before. Here's why. I still think most people aren't using any product like ChatGPT or Cloud or Grok at all, really.
And they don't really fully know how to use it yet. Yeah. And the second thing is that I don't think anybody has any switching costs yet. Agree. There's an early life cycle of any new category. Where for anybody who gets even marginally interested, you're, you have this ongoing conversation where you're like, which is the best one and what should I use?
And so like, you start by opening up the app store and you download a mail client. And then you're like, I wonder if there's a metal mayor client. And like, for the first couple of years of the app store, You probably download two or three or four mail clients and maybe you end up [00:05:00] at Apple Mail at the end like you, which drives me crazy, or maybe you end up using something else.
But before the incumbent is really like set in stone, there is this like jump ball period. This is what CNET used to live off of and all the tech media outlets is like, this is what coolhunting lived on back in the day, and dot com is like, a new category emerges and somebody needs to write reviews where they're like, I don't know, which of these three pieces of software should you use?
And I, I still think we're in that phase. If suddenly, You were consistently 10 percent better than everybody else. I'm just thinking forward to the 5 million, 10 million, 50 million, a hundred million, 400 million conversations. Like I'm just thinking of as 8 billion people come in to use these products, they are continually going to have this conversation where they're like.
Oh, I tried chat GPT today. It might be the first thing that they try. Sure, because it's the best known thing. But then you're going to go talk to your friend who's the chat [00:06:00] GPT guy. Like you're, you're not going to do it alone. You're going to go find the guy, you know, the woman, you know, who's like played and is like, Hey, is this the right one?
And they're like, Oh yeah, yeah. There's like four guys that do it. There are four companies that do it. And the one that I like the most is X.
Fraser: And
Nabeel: so you don't have to be 10X better. When the consumer base is comparison shopping before they've settled in once they've picked their toothpaste, like you're not switching them off their toothpaste, but when toothpaste comes out for the first time, you go for this through this period where you're like, I don't know what kind of toothpaste am I supposed to use?
Maybe I should try three of them and decide what flavor I like. And so even just like having a marginally better flavor gets you there. I think the assumptions that we have that are different. Is that even though and what was announced this week, you know, the number of users that are using chat to BT and opening eyes case, I still don't consider them the incumbent yet.
And so I think the model you're using, which makes sense to me is the startup attacking the incumbent [00:07:00] kind of model, and I'm just not even sure we're there.
Fraser: That's fair, but I think the thing that I was. Assuming is that a 10 percent better here was constrained to the model. And I think that like so much of my world view is still model first, and I just can't get my head around the population carrying about a 10 percent delta in the quality of the model.
But I think you're saying that the product experience, if something is 10 percent better overall,
Nabeel: let's be clear, I'm not talking about 10 percent better on evals. Right. I'm talking about, I get it, 10 percent better to a person using the product. And another example, like the analogy is it's like, we're a year into search engines.
We're still five years before Google, right? Yeah. We're six years before Google. It is AltaVista, Yahoo, you know, ask Jeebs time. And I'm just remembering in that time period, everyone who was trying the first search [00:08:00] engine, it was a viable conversation on a month to month basis to be like, Which search engine do you like more?
And, and if one had consistently been 10 percent better than all of the others. They would have won up until Google wiped the field. I don't know if we have a Google asteroid coming in five years or whatever, but do you see what I mean now? Yeah, yeah, kind of, like, because
Fraser: Google was, like, 10x better. And so that's why, like
Nabeel: Google was just unequivocally better.
Fraser: Yeah, yeah, yeah. I think I kind of get there if I change my frame of reference away from thinking of it as the model is 10 percent better. And maybe, like, the really cold way of saying that is Uh, I was thinking about it like 10 percent better on evals. No human is going to care about that, but you're saying, yeah, let's, let's switch.
You know me,
Nabeel: Fraser. Like I, I, I don't care about evals. Like it is 10 percent perceivably better to a customer, right? Like a. Customer couldn't explain maybe even all the reasons it's better. They might muddle through it and also consistently if they're 10 percent [00:09:00] better and then their competitors 10 percent better a month later and they're another different competitors, 10 percent better than if it bobbles around, then people will just kind of go to either the first thing they started with or they'll just go to the default, most popular thing over time.
Um, but if there's some model of some company that is giving you chat experience, which is, I do think a new affordance that you'll just use every day and they're doing it in a way that is consistently marginally better than the competition, I don't think you need to be all right. All right. All right.
Fraser: Yeah, I got it. You know why I got it as well? Because I'm going back a handful of conversations now. My my worldview is that this is just a new behavior that we're all going to have to adapt to. And Like that's going to take a long time, and part of that adaptation will be figuring out what's the right best product, and over a long arc, something that is 10 percent better should win in that world then.
Nabeel: It's a long race, and more importantly, you're comparing, like, I think the really [00:10:00] important thing is most consumers are still comparison shopping, and will comparison shop, because most of them haven't even started using these products yet. And two, to go back to the beginning, there's no lock in yet. There is a world where you've uploaded enough documents, you've told it enough things about your kids, you've started to build some real rhythm with this thing where there's just real lock in, then switching costs become real, and then the kind of B plus product will do just fine if it's the incumbent.
But I'm just not sure we're there yet, and I, and I wouldn't. I wouldn't cede the ground just yet. Everybody likes to call the game over. You know, everybody, everybody called the game over the week after DeepSeek installed all their NVIDIA software. Like, everybody loves to call the game in the first quarter, but that's just like not how this works.
These are, these are wars of attrition often.
The questions we are asking
Fraser: Yeah, that's like a very natural transition then to topics that we've been thinking about and discussing as a firm as we think about where we are and looking forward to this coming year. One thing that's been on my mind is we talked about it briefly on on [00:11:00] a previous episode was Kevin's presentation from Spark around the early web, who were the first movers and how did they do and then who were the ultimate like quote unquote winners of the space.
And I've been thinking a lot about a second mover advantage and like, what is the chance that we're really just seeing the interesting markets illuminated by people who were quickest to pull, you know, a good new capability into them, whether that's law or code or what have you and. You know, I think there's a, there's a high likelihood that some of these will be won by the first mover and there's going to be inevitably a lot of new companies that are starting today that are going to win markets that we think, you know, are already like one, so to speak.
Nabeel: Yeah, it's, uh, it's, in fact, we just talked about it, right? Like, we just used the search engine example from early web, where you have this massive fight, you know, between AltaVista and [00:12:00] AskJeeves and everything over search engines. And then it turns out that everybody, you know, needed a couple of years to digest it all, come up with a new novel thing, and then Google comes in and suits the field, uh, a little bit later.
So that, that is a, we already referenced a very obvious one. You know, one way of thinking about this is I think about whether there's first mover, second mover advantage in a market. And let's just talk about which markets, right? Like, so where do we have real traction today? We have real traction in AI coding.
Those companies are, are taking off like crazy. We have real traction. There's a lot of law, AR startups. There's, um, a lot, anything that is voice. That calls people seems to be doing quite well right now, like making phone calls to humans and then charging other people for those phone calls to do some kind of AI thing.
Those seem to be working. We could go down a list. An easy way to tell what seems to be working is to look at the Y. C. Class and look where they're centered right now. So you could look at the growth rounds being done, but it's also for some reason it's completely mimicked in a Y. C. Class, uh, where, of course, founders are opting in and [00:13:00] being like, well, that looks kind of good.
I'm going to do my thing. That's 5 percent different than that. You know, I guess the question is. Where are there places where it's important to look for second mover advantage and wait or search for something deeper? And where is it? Go time, right? Where do you like, do you feel the heat and you feel the run and you should be out there in the market with something and learning with your customers?
How do you decide where you do one versus the other? I
Fraser: wish there was a tiny way to answer that question. Where when there's a real differentiated product experience that matters and is valuable, that probably is a case where the second mover can do very well.
Like Apple is famously the last mover in the markets, right? And they delivered the right product experience and have shown time and time again that That matters. And so in many markets, the right product experience, which is going to be a combination of the product and the technology. And here we're like super early in the underlying technology continues to improve dramatically.
And [00:14:00] so does the new reasoning models introduce new capabilities that the previous products can't even absorb in like a very native way? Into these markets, I don't know, like I, maybe, but maybe not,
Nabeel: right. I think it's the second mover advantage, like where I'm trying to imagine being on the board of a company or talking with a founder where your advice would be like, don't go too hard right now, even though there's revenue or traction, like it's not the time to go in or go all in.
And for me, in a way it's, it's somewhat ignoring the revenue numbers or the hype numbers or the markup numbers in the industry and trying to realize. How broken the real solutions are, because we did already go through a wave where A bunch of people, customers really wanted to spend tens of millions of dollars on a bunch of very early AI products at the beginning of chat GPT that had huge spikes in revenue and then dissolved.
And so, you know, for me, the second mover advantage thing just comes out of, are they fully solving the problem or [00:15:00] not? And I understand that's a, that's even, that sounds simple. It's still hard because of course, like we're the business of early stage investing and founders are in the business of starting something.
It's always broken a little bit broken in the beginning. It's always like. Not perfect. And so none of these things fully, fully solve the problem. You're trying to set marks and problems that are worth working on for a decade of your life, right? It's not going to get solved immediately, but it's like applying the amount of fuel and the amount of certainty.
That is mapped to how much you believe in the thing. And so, a good example for me is, if you see five competitors that are relatively undifferentiated and all doing kind of well, but customers are already doing bake offs between them. Mm. That's a sign that one, it'll be hard to compete to the cost might go to zero, right?
And three, if they're switching it, yeah, it means nobody's doing anything that satisfies that customer fully anyway. And so maybe it's worth being more differentiated in those worlds that that might mean that you go after a different customer segment.
Nabeel: It might mean that you [00:16:00] try to solve the problem a different way, but it's like dig deeper. You know, the harder thing is if you're a founder, that's one thing. If you're starting a company or you're looking at the market and you're like, should I start another law startup? You know, it's, it's harder when you've already started the company and now there's five competitors and you're looking around and you're trying to make the really hard decision that actually.
Now you maybe weren't a commodity six months ago, but you kind of are a commodity now. And like, that means that you're not going to increment your way to the future. You need to like go set some mark right way ahead. Yeah. Uh, and meanwhile, by the way, because there's five of you, you feel like you're in a dog fight, you're hitting, trying to hit revenue marks for next month, like, and there's revenue to get, that's a very hard founders.
I've seen a few founders really do that really well, but we had granola on last time. And that's a good example of. Looking in a market that looked undifferentiated. Meeting transcription was a solved market. There's five people that have all raised tens of millions of dollars and blah, blah, blah. And then being like, I don't think any of these people actually really solve the problem.
That's a perfect
Fraser: [00:17:00] example where there was a clear new capability that allowed you to, you know, quote unquote, solve a problem. Right? Transcription, but it has taken real great product work to like show that there's differentiation in delivering that capability to an end user.
Nabeel: So let's move on to another one.
But, but, you know, just to back up for a second, I like the spirit of all this. We're not a thesis driven firm. The founders come up with theses and, and we're there to partner with them when they come up with amazing theses and try and help them along the way.
So we're not a thesis driven firm, but that doesn't mean we can't. Have a prepared mind and we can't ask curious questions. And so, you know, big picture is every year. And then sometimes we, along the year, we come back to these questions. We kind of ask ourselves, what areas are we curious about? So this is not a, which markets are you investing in? Yeah, yeah, yeah, yeah, yeah, yeah, totally. This is more of a, what questions are we asking?
Fraser: Yeah, I mean, to add credibility, to add credibility to that, the last [00:18:00] investment that I did, unannounced, so we won't share, I happened to go see them on a Wednesday.
I came back and I'm like, you got to come with me to see this company tomorrow. And we drove and saw them and you know, yada, yada, yada. Very quickly, it all came together and it was not something that was nowhere
Nabeel: on this map of questions that we're asking or anything like that. We love a good surprise. So, but it is worth sharing.
Like, look, we just, this is supposed to be. An extension of the conversations we're having internally, and that's really the spirit of what we're trying to do here and to bring founders and others into this little process that we, this muddled process we're going through in AI right now. So I think it's just worth, you know, translating our internal conversations externally to the questions that we're asking.
And so, second move your advantage is a good first one. Um, let's take one more of the questions that you put down internally. I have them in front of me here, and then we can take some of the questions I've been asking internally.
Fraser: I've been spending some time just really appreciating what has been done with the reasoning models.
And I think [00:19:00] I've said this to you now. countless number of times. But the surprise of last year for me was how quickly 03 appeared after 01. Like 01 totally pointed to a future of 03 and I would tell you about how exciting that's going to be and I can't wait for some number of years when that arrives.
And then you woke up the next day and there it was. I think that we are seeing that there's an entirely new vector for training models that is going to Unlock a whole lot of different use cases for product builders. I also think that the thing here that is maybe underappreciated is the amount of compute that's required for this is less than like a pre training effort.
And so I think we're going to see a lot of different academics as well as like hobbyists being able to explore with this type of post training. And I think that we're going to see people shape products. Uh, with the hosted reasoning models like 03 and others that are coming as well as being able [00:20:00] to train their own off of llama.
And then the question is like what, like deep search and all of the derivatives that have then come up are so obvious, like we've spoken about at length that that research and synthesis is like a beautiful use case for these models and that reasoning now is able to deliver that in a very beautiful way, but like, where else are we going to see reasoning models applied to products?
I think there's going to be a lot of beautiful experiments in this area and that we're going to see significant stuff in this next year. Yeah. And
Nabeel: more importantly, like I, I love this because more importantly, it's saying like, listen, we all tune ourselves very quickly for pattern matching, what's going to work and not work.
And it's just acknowledging that the solves for reasoning and deep reasoning, the things that might Might come out and be new opportunities because of reasoning might be different. Like it's a different sort ordering stack than the things that might have come out of, say, a good agent or [00:21:00] the chat GPT or GPTs previously, even if you just take certain vertical markets for a second, legal summarization and transcription as a company, that's a great 2024 2023 company.
There's an entirely different company you would build. Illegal if you Are assuming that reasoning is your differentiation versus a summarization or transcription as the effect of the model. And so, you know, there you might, I don't, you might do things like I want you to identify subtle contractual risks in this contract versus today's Harvey's and so on are like, just summarize the contract for me, like write a brief, right?
Or I want you to predict. I'm coming off the cuff here, but like in legal, like I want you to predict how this clause in this contract might interact in future scenarios in some weird way, like draw me parables until I can understand why this might go wrong. And so, yeah, it is like. A reminder to us to sort [00:22:00] order differently based on reasoning because different things and different companies and different ideas might rise to the top.
I'm also curious on this side to just instead of taking the market view down where you're like, Oh, how will reasoning apply to legal or health care or finance or education? Kind of the reverse side, which is like, Hey, what are the, what are the most deeply reasoned areas of the world? So forget market down and use case in like.
Just like what are the places in the world where we just apply incredible amounts of reasoning and then what does this feel like to work from that way in and another way like an area I think about that is areas like to use the law example, like I'm paying attention to the Supreme Court right now, like constitutional law is like there is no hard, fast answer, right?
A lot of times it's literally like an interpretive principle. And so trying to look through many, many layers of a question and. Um, I don't know what the business or the startup output from that [00:23:00] is. I wouldn't jump to that conclusion, but it's an interesting situation where you're like, Oh yeah, that is an area where reasoning really, really matters.
A lot of game theory stuff, either in economics or elsewhere, strategic decision making involves like lots of like reasoning around what the rational actors are going to do in a situation. And economics uses. All kinds of really simplistic principles to try and come to those conclusions because they haven't been able to build off of right, you know Reasoning agents until this year.
So what does that mean for the field of economic that kind of stuff? That stuff's fascinating Only questions right now, which is going to be the nature of this entire podcast will be very frustrating to people Is we're not giving you answers. We're asking good questions, but I I'm fascinated with that I
Fraser: agree the interesting thing for me as well.
Um, like a meta point is that We went from GPT 2 slowly to GPT 3 and like that arc slowly unfolded and we could have time to experiment to figure out how this new capability can be brought into products. And I think we just like have smashed into an exponential curve that's very different with like reasoning.[00:24:00]
And we're seeing that it is. Why is
Nabeel: this any different? Are you sure? Why wouldn't this just be The same thing that we went through before, which is like everyone's going to do the dumb low hanging fruit thing first that involves reasoning, it will be wrong, and it will take 18 months for it to really be thought through and internalized and productized and turned into a real company.
We
Fraser: are seeing everybody do the low hanging fruit first, right? Like, all variations of deep search or deep research are the obvious first thing to be doing. But the difference here is that it's not inconsequential, like this is a, this is a profoundly new product experience that's adding value, like in, in a lot of people's lives.
And so I think the difference thing, the difference is that you're not seeing people like. You know, the first version of GPT 3 was great for a fantasy role playing game because all the limitations were great for that. And then it did simple ad copy. And now it's writing your high school essay. We have just jumped to the point where these reasoning models are great and that they're [00:25:00] going to become great this year already in many new products, undoubtedly.
Nabeel: Yeah, because they're standing on the shoulders of giants thing. They're stacked wins off of the previous work as well. Yeah, for sure. Like, absolutely. Like that's another aspect to it. For sure. Can we sidebar here? You're a fan of deep research. You use it a lot. You're used it. Do you find much differentiation between the various deep research products?
Like I'm, I'm sure you've used perplexities, deep research, and you used opening eyes and so on. Do you, do you find much difference? Which one you'd use for different reasons. Uh, the honest
Fraser: truth was the, it was perplexity integration of R one, the deep seek version that, you know, I came running to you and I'm like, Nabeel, you have got to try this.
It is crazy. And that is, that is in the, the evolutionary like lineage of those things that followed. It was just more reasoning, deeper search, which is to say like. No, like they all feel like of a kind. I think if you're doing like very [00:26:00] sophisticated, nuanced type of problems, the thing that has access to O3, the better reasoning, no surprise, is better than the alternatives.
But if you're asking like, what What USB cable am I going to want to hook up to for my Mac? You know, my Mac mini, the reasoning in the search is pretty amazing across these things. Yep. I don't know. Do you, do you notice a difference?
Nabeel: You've been playing around with all of them as well. I love trying to understand the nuances.
I think one of our first podcasts, I was talking about like, when do you go to perplexity? When do you go to Google? When do you go to Chachapiti in that world? And trying to work out rubrics for that, which feels very clear to me. Um, although it's changed a little bit over time as these guys have moved.
But no, I don't, I don't. The thing that I found clear is there's still writing style matters deeply. Like, yeah, I don't care what Google Gemini's research product is coming back with because the writing is so bad that I just don't want to read it. I can't like my eyes start. Glazing over when I [00:27:00] try to read Gemini's prose, and so you have to get above some bar, which is actually not easy to get above of readability and interestability, no matter what the reasoning is inside of it.
But perplexity is there partially because DeepSeek and OpenAI are there. I don't know when I would go to perplexity deep research. Versus OpenAI's deep research. I would suspect that OpenAI is doing something at a much deeper level. So maybe if I had some like internal, uh, lever that was like, this is really hard.
Think about it more. That I might go to OpenAI just by, but I don't know, or my, or just my default might be there because I'm there for other reasons or something. I'm not sure. And then obviously the other competitors will come out with their work. It'll be interesting to see if Anthropic releases a, Deep research product or somebody else, what they do and what that means.
And the kind of second iteration of this. Yeah, the thing that feels like it's missing with deep research [00:28:00] for me is access to more context and data. It's weird to me that they released this product, and I get that it's going to go out and read things off of the web, but it's still weird to me that they released this product without a kind of notebook LM style.
Why don't you go grab these 15 academic journals and toss them in here or why don't you give me all the internal PDFs that you also would have read through for research. Let me synthesize that with internet data and give you back stuff. I assume that will come.
Fraser: It'll come. I, I, I, you know, I mentioned to this to you earlier where I feel like they've gotten a little bit of their groove back.
Like I love the fact that they released it without any of that stuff, right? It's here, here is a research preview. We are a product in service of the model. There's as minimal like product experience built around it as possible. Let's just like get it out there and see what we can mold from there. I love that.
And so, like, yeah, I think all of that stuff will come, whereas, like, if they launched it and this wasn't a response, they wouldn't have had to have [00:29:00] built out all of that stuff, and, and now they, now they meander the, the IDMAs, and, like, they have users, and usage, and, like, feedback to help guide them through that.
Nabeel: Okay, so let's go to next questions that we're asking for 2025. Let's go to next questions we're asking for the
Fraser: year. There's, like, a natural bridge from one of mine that we just talked about to one of yours, and that is, like, If reasoning was a new capability, but it accelerated in terms of how good it was so quickly that we're going to see these profound products soon, computer use is another new capability that you've been, you know, mulling over and asking questions of.
So why don't you talk a little bit about that? Yeah, and for
Nabeel: contextual background here, we did invest in Adept, which is trying to build, originally trying to build its own model against this. So I've been looking at this space quite closely for some time. OpenAI obviously has released their operator computer use, Anthropic will have computer use, like it is a area that [00:30:00] feels the way that reasoning was last year.
It feels like it's 12 to 18 months to turn it into real applications now that there's APIs available. But also at the same time, to be, you know, slightly skeptical, we've had some measures of research oriented, maybe not available to the general public, but research oriented computer use for a couple of years now.
And so part of me would be skeptical to say like, well, if it was really obvious, why wouldn't it have, have appeared right now? Um, but the other part of me just watches using these products and just feels like they're, none of them are quite productized well enough yet. You know, they're not perfect.
They're okay. 87 percent of the time or 92 percent of the time or 96 percent of the time. And unlike trying to tell a story or do an RPG dungeon chat, GPT experience, that variance is a real problem. Yeah. And so the question is like, what, what are you going to use computer use for? I think there's probably two threads that I'm very curious to go down.
One is, do [00:31:00] these reasoning agents and probability agents help these computer use models? Is there a way that that might help it get better at understanding when it's about to screw up and go ask for help or reason around how to fix it itself? You know, call your boss in and say, I'm not sure if I understand how to scroll through this or whatever.
And then the other thread is, what are their areas? And I have some sketching on this that I've been doing, but like, what are some areas of the world where that variance is okay?
Fraser: Mm hmm.
Nabeel: And being a pixel off or five pixels off. Is okay. When people think mostly about computer use, they think about RPA, RPA, like applications.
They think about, I want you to go to this website, click on these five buttons, scroll, copy, paste, that kind of thing. But one place where being a pixel off is maybe okay is, you know, playing a computer game. The way that you can play a computer game every time you do it and the way you go through things, it's not even deterministic in many [00:32:00] cases, especially with social games.
Now, what's the business or startup idea there? That's a whole nother question. But as a thought experiment, like, what are the areas where, just like the process we went through with early GPT, what are the areas where The randomness is okay, or even ideal. Yeah, what's computer use going to be? I don't know.
I've been thinking about it for a long time. It feels like in 12 months, we're going to have an answer because it feels like we're finally getting to the point where these things are getting to the public. Yeah. They're getting exposed. I think enough startups, frankly, are trying to play with those APIs and are really pushing on it.
Fraser: I think this is the opposite of reasoning, where it's a brand new capability that requires As you said, great performance and reliability in many use cases for it to be good, and it's just not there yet. And so, like, we will go through the equivalent of a GPT 2 and a 3 and a 3. 5 and, like, all the other arcs as we figure out the use cases, and we'll wake up in 12 to 18 months and there will be [00:33:00] a lot of profound stuff.
I would be surprised if there's great value delivered like a deep research with reasoning in like the next month with these computer use products. I just would be so surprised.
Nabeel: There's some like little thing inside of me. There might be an RPA company here and it might be great and huge and that might happen and I'm certainly open to it.
But there's something that smells like the thing a couple years ago we were trying to have. The first versions of ChachiPT be agentic and run around and do things and it just would go off the rails and be terrible and it just wasn't good enough. Yeah. And it turned out that treating it more like a co pilot was a good idea or evaluating.
It's better at evaluating than writing, you know, back then it's like I could give it a piece of, I could say, please write this in the, in the style of Paul Graham. It's terrible if I gave it a piece of writing and said, how different is this writing from Paul Graham? It's actually quite good at analyzing text than writing text in a good format.
And I think there might be something here that's like that, where, hey, [00:34:00] just because it knows how to work a computer, the answer might not be that it actually does the work on the computer. The answer might be that it like, maybe it's watching. A worker do something and then like reaching in every once in a while being like, you're about to do that wrong or like you seem confused there.
Can I help in this one spot? And because it understands the language of the world that you're in, it can come in and assist, but it's actually not trying to do 35 actions in a row. autonomously, because we're just not there yet, like it's not ready to be Devon. Like everyone loves to have an AI engineer go and run a code for five hours, and we probably will get there, and there's a reason to maybe think about trying to get there, and maybe you try to be early.
But like, I think computer use is more in the soup of like, you know, we're in the GitHub copilot phase, not the Devon phase. And yet, most people aren't articulating or trying computer use in that context.
Fraser: Yeah, that feels good to me. Like I can, I can totally imagine it. We have to go through the co pilot step of just like cheap code [00:35:00] completion before you can get to like the miraculous thing where it's doing crazy automation for you.
That feels good. There's a way to tie these two things together, like Bob McGrew, who is a buddy and was former chief research officer at OpenAI, had a tweet around OpenAI's deep research, and I'll just read it. The important breakthrough in OpenAI's deep research is that the model is trained to take actions a part of its chain of thought.
The problem with agents has always been that they can't take coherent action over long time spans. They get distracted and stop making progress. That's now fixed. And so the interesting thing is that computer use may actually be something that you and I interact with through our products on a regular basis, but indirectly because it's the model calling it through its chain of thought.
Reasoning process in order to go and get the information that it wants to help us, and we aren't even aware of, you know, it's doing of that.
Nabeel: Yeah, yeah, I think that is actually a great reconnection.
Fraser: What else we got? You want to do AI as Muse and not an Oracle? I [00:36:00] think that's a great question. Why aren't you seeing more Cursor for Axe?
Nabeel: Yeah. How can we build more AI tools that enhance human thinking rather than trying to replace human thinking? The AI is Muse and I'm Noracle kind of phrasing. I think that is because Silicon Valley is broken, and people are lazy. That's all. That's just a simple put, huh? I think, look, people, I have this phrase I wrote down, which is like one of these like mantras for yourself, for me, which is just like trying to nudge the world.
Into taking creative risk over arbitrage, and I think we have an engineering mind instead of an artist's mind when we attack many new problems when often. The more joyful thing to build as a company. And frankly, they think consumers like more, frankly, the larger potential outcome as a company often is not [00:37:00] efficiency oriented.
And I understand that like engineers and economists try to run the world and all they know how to do is to walk in and say, well, if you just took the profit margins from 12 percent to 14%, wouldn't you be in a better shape? And that's a lot easier to think through than inventing a new world. And so I think it's all of that thinking baked into here.
It's a lot easier to pitch to a VC that you've gotten efficiency gains. And so if you're just trying to pitch efficiency gains, you're going to try and pitch a customer and you're going to say, well, Morgan Stanley was taking five minutes to do this job before. And now it takes two minutes. And so like, that's, that's the same mindset that, that drives you towards arbitrage.
The thing about arbitrage is that somebody else is about to arbitrage you right after that. Right. And when you really truly invent something new, Um, or if you invent something that people are doing the joy for it, it's very hard to replace. And, and so those areas of, of the world have always intrigued me more.
And I think that used to be an area where Silicon Valley was very, [00:38:00] very focused. The kind of like Apple is a liberal arts mm-hmm . Oriented company as much as an engineering oriented company pitched that jobs used to do. I think in this more recent. Machismo, efficiency oriented world, we get a little bit less of that.
So anyway, that's a sidebar rant, but I think that that's why we're not seeing it. I think we're not seeing more tools for thought that are AI is amused on an oracle because we are sitting in a cultural pocket that is very efficiency and arbitrage oriented. But I think that's giving up the larger goal.
Like a really good example of this for me is exactly what happened in diffusion models. Thanks. You had every single attempt in making new art moves, you know, as we got to fusion models able to make art and you had the mid journeys of the world, you had the dollies of the world, you had the Leonardo AI's of the world and, and I remember all of them pitching that same first year and.
You [00:39:00] know, DC's got very interested in Mid Journey because, of course, they could see the revenue and they got excited. But all the advice is bad to what they're supposed to do. Hey, when are you going to go talk to Paramount Pictures or Vivendi or Blizzard or some game company and make art for them and help them, you know, look at how many production artists are on this game and you could make it more efficient and you could like, it was all an efficiency arbitrage play.
Because that's the way we process the world. And I give David a lot of credit at Midjourney and it was the right pick. You know, I'm not going to go work with the old world and try and make them a little bit more efficient. This is going to be its own thing. People are going to do this thing for the joy of making the art in this world.
I can't size that TAM for you, man. Like, I can't build that TAM slide. And that's okay. Because I'm just going to do the thing I believe in. And so they have, I would consider what I was almost like a net new thing in the world. I don't know, you know, there's lots of questions to answer when it comes to what is AI [00:40:00] even as a Muse versus an Oracle.
Right. Cursor, I think of as that way. It's not trying to be Devon. Yep. It is also not trying to be Copilot. It is Your partner, it's going to go off and do 30 seconds of work, not 10 minutes of work. It's going to come back and ask you questions. It's gonna, it is like your little partner for coding. And I would love a little partner in most of the activities that I do that have some creative element to them.
And so what is the cursor for X sounds like the stupid VC language version of processing this. But I think I mean something a little bit more profound. Like I, I I love the interactions of coding with Windsurf and Cursor and that, that level of, and I have no desire, although I know the world wants a desire for something to just be an AI engineer to go off and do all the coding for me.
Right. That should exist. It's less interesting to me.
Fraser: Yep. Yeah, yeah. I heard Satya on the Dwarkesh podcast say that like, There's white collar work and then there's white collar workers [00:41:00] and that white collar workers are going to continue to do cognitive tasks. They're just going to change, but the actual white collar work that they do may look very different.
And the reason that I bring this up is, like, that just resonated. Like, I think that we are going to continue to be in a place where there are workers. And in your Muse example, It is white collar work working with AI in like a new way to change the way that like software engineering has been done. And it's not, it's not, it's not that AI is going to get rid of the white collar worker, the engineer.
It's just going to change the way that they do their work.
Nabeel: I think there's lots of embedded, really incredible questions to ask when it comes to what are those tools for thought in the future? Like, like in a creative field, like mid journey. What are the types of affordances and UX's that you want for a user as they're trying to walk a solution space that is like trying to get something that's inside [00:42:00] of their brain outside in the world, but it's like there's no English language for it.
If you're trying to describe a song that you want to exist or a poster that you want to exist or a painting that you want to exist, there just is no real perfect language. And so how you navigate it. through the like sea of possibilities with an AI to get to something that is kind of like what's in your head or more likely, um, that the back and forth with the AI ends you in something that you didn't imagine in the first place.
Like, right. And that's a little bit different from. Less creative fields and more analytical fields areas where you're trying to get to a solution, you might not know how to get there. And so asking really good questions as you get there will help you get there. That feels like two wildly different product areas.
You know, analytical, I'm trying to figure out the truth of this company and whether retention curves are working well, or whether we have product market fit is an example where like, that is actually like a copilot II thing you could try and figure out, do we have product market fit right now? [00:43:00] It's a creative exercise, but you are trying to get to an answer.
And obviously the arbitrage version of that, the Silicon Valley arbitrage version of that is, Hey, we can write your SQL queries faster. But like, that's just like, that's not interesting. Like that's not getting to the root core first principles question that a person is trying to answer there. The white collar question, white collar work question that somebody's trying to answer there.
Yeah. Yeah. I have lots of questions there. I think it's, it's, it's super interesting. And it's also an area of founders or are navigating it that there just aren't going to be 35. Other precede companies doing the same level of investigation because of the air we all breathe right now, which is very arbitrage oriented Yeah, well put did we talk old markets at the beginning?
We talked old markets at the beginning, right? No,
Fraser: we didn't we talked about it as something that we could talk about
Nabeel: Yeah, the last question I'd ask that is on old markets, then let's end on that one. I think we have other areas of curiosities for us internally. I try and constantly update a list, [00:44:00] but there's a handful of folks.
And I actually hope this, this episode leads to, you know, maybe I'll listen to this and you think we're We should be asking different questions or you have a, you have an answer to one of these things. These questions we're asking, maybe you'll have three other areas you're curious about and we'll do next week on what anybody else is asking questions about right now.
That might be fun to explore as well. So yeah, basically like which, which legacy markets are ripe for AI reinvention. And obviously this is a question that everybody asks, but I think the general lens with which people look at is like the last few years they're looking at the thing that's like. The unicorn from four years ago that they might be able to reinvent.
And so, yeah, the, the area I have, I am drawn to curiously is, is what are the areas that kind of are almost always reinvented there's some truth to. Things and industries that are always on the front end of innovation and so kind of always get it reinvented whenever there's a paradigm shift. [00:45:00] And so this is things like we talked about the instance of like discord going back to AOL.
I am going back to IRC like messaging just seems to get reinvented with every new paradigm shift marketplaces task management. Review systems like Yelp, each of these just seems to kind of like absorb, I don't know what it is. We probably could invent some academic phrasing for why these particular industries, if we're trying to write an HBS article or something like that, a Harvard Business Review article.
Right. We, we, we, we, why these particular industries always get reinvented, but they kind of tend to, and so. Are those the areas where I'm just trying to be present and realize that even if there isn't a startup coming in and pitching this week that they are likely to be upended that we still haven't answered really basic questions like which restaurant should I go eat at tonight or how do I arrange my tasks for the week and these are kind of like in a way.
Interminable questions like we'll never fully answer. They'll never be the perfect answer. And so [00:46:00] they can always absorb new technologies come up and better answers. So, you know, again, it's a question. I don't have a great insight into it. The whole point is like just being curious about it, right?
Fraser: It leads to more curiosity and more questions for me.
We might have talked about this before, but like a lot of these things have to be re imagined for a world where the previous business model also isn't as feasible as it had been, right? Like, Yelp reviews made sense because of the social exchange that happened within that community. And then the business worked because of ads.
Both of those feel like in a world where your, your AI assistant is providing the recommendations and the reviews somehow. Like where, where's that coming from? What, what's the engine that, that makes that whole thing work?
Nabeel: Yeah. I mean, I think that it's, it's almost like we talked earlier about some themes.
Like being able to do reasoning or another theme is just this idea that I've been talking about at least lately, which is like if Web 2. 0 was the wisdom of crowds, then this age is really like the wisdom of experts. You're not [00:47:00] trying to get the average of what everybody would solve a physics problem.
You're trying to get how a PhD person who's absolute, absolute physics master would solve this physics problem. And so that's a very different paradigm. Taking summarization is another paradigm that comes out of this. That's an old one, but it's another one that comes out of this paradigm. Computer use might be one too.
It's taking these paradigms that emerge. Maybe we should do an episode on the paradigms that have emerged. That's actually a future episode would be how do you, which lenses of AI do you apply to each of the things. But that's exactly right. It's like, how do you look at each of these things? And then apply that paradigm to it.
What is this new world we're in? Yeah. And what comes out of, you know, another one is malleable software. Like we can now write software and change software. So for instance, every messaging application, it's for me, this is a perfect one. Like every messaging application has a left bar, which has some levels of categories on it.
It's either oriented by the people that you're [00:48:00] talking to, or it's the channels that you're able to communicate in, whatever. They all have some fixed ontology. Why would they have a fixed ontology in a world of malleable software? Like, why wouldn't those things rearrange themselves dynamically? But what does it mean to build a messaging platform from first principle that is meant to be that way?
It's probably not a thing you slap on later. It's probably a new kind of platform. What does that mean? Right. Like you said, What does it look like? Yeah. What does it look like or feel like to use? And what promise, new promise are you making to a consumer? Yeah. Yeah, that, that you could do. You could go down the list.
You know, what does it mean when you're recommending a restaurant? And does that mean that I get to pick the expert? I want to know what somebody who really knows this restaurant is. Is going to pick for me and it should be at this point, ideally one shot, three shot. You should really know if it's an AI, right?
I shouldn't be glancing through a bunch of photos and a bunch at some point, maybe I'm training a model on my own restaurant recommendations. I don't know what the answer is, but yeah, I also wonder where we even get new [00:49:00] data. This is a totally different subject, which we, you know, it was like. I don't even know where we get restaurant reviews in a world where the wisdom of crowds model is fundamentally broken, right?
If the ad model is broken. Then there's like, the principle promise of the internet is broken at this point, right? The idea was I made content for free. That gets served from the search engine. You click on the ad and you get exposed to the thing, so that's the virtuous circle of the internet. That's broken.
Fraser: Like, I used to go, like, what do I go on to watch? I'd go to Rotten Tomatoes and I'd go page load, page load, page load, page load, page load. I recently asked Claude, and actually it was, I haven't told you this, it was, it was awesome. I'm like, here's what we feel like, here are, like, the temporal aspects of the shows that we want to, like, capture.
Right. Give us a recommendation. And it gave us a list of three and we read one and it sounded awesome and we went to like of all places peacock like it was on some like, you know, and it was good. It was good. And we didn't go to Rotten Tomatoes. We [00:50:00] didn't go like we didn't give them 25 page views. They certainly probably got their information from sites like that.
But where are they going to get that in the future?
Nabeel: It's very, very, very curious. And you see little bits of it starting to emerge, right? Reddit is selling their data. Does that extend back to the user? Does the user of Reddit now get an extension of that, of that fee? Or do you still contribute to Reddit knowing it's going into a model?
Is there a new version? Is it just Yelp settling their data? Or is there a new reason why you might contribute to a Yelp in the future to help inform a model, to help inform some other user in the future? Where we get our data from and how is also like an interminable thing that'll be worked out in the next five years that nobody knows the answer to, because certainly the Web 2.
0 era paradigm is broken for now. And some of these ideas of old markets being reinvented, it might literally come from that. It might be like, Hey, we figured out. How tasks get done or a new version of task management or a new version of a marketplace or a new version of reviews because we [00:51:00] have figured out this new economic model because we have this by wheel new economic model that leads to a different kind of experience, which also leads to a new revenue stream and like, I don't know, I don't know what comes out of that.
Whatever is broken often is the first step to leading to a new solution.
Fraser: Yeah, well put. Mhm. Well put like the answer isn't it for the next number of years, it's going to be Reddit. It's going to be all the places that already have like a network because those network effects are strong and they're going to be able to sell and make like good revenue from that.
But that's going to diminish with time. Like it,
Nabeel: yeah, those things feel like FM radio in a way like that, that, that's right. It feels kind of valuable, but. It feels like somebody is going to build a new market that incorporates and thinks through, not brute force, I'm just going to recruit a PhD to answer the question, but like, how do you create a real new, a novel market structure?
Yeah. That'll be fun. Good conversation, man. Well, I'm not sure we'd be done. Yeah. Thank you. Thanks for chatting. We'll figure out how we package all this up and make [00:52:00] sense out of all these questions we're asking.
Fraser: See ya. See ya. Take care.